
From left: Moderator Rachel Mercado of PriceWaterhouseCoopers;Carla Leibowitz of Arterys; Michelle Marlborough of AICure; and Gary Seamans of IDx
If the ongoing discussion on machine learning, deep learning and other variations on artificial intelligence has taught us anything, it is that despite the advancements in AI and potential to shape clinical decision support, computer technology is only as intelligent as its programmers. It’s fallible. But perhaps one way to defuse the tension and angst around clinical applications of AI would be to get companies to talk about the safeguards they have in place when their machine learning algorithms fail to accurately interpret an image or reach a conclusion.
A group of health tech entrepreneurs talked about how their companies address machine learning algorithms when they have an off day as part of a panel discussion of AI this week at MedCity INVEST in Chicago.
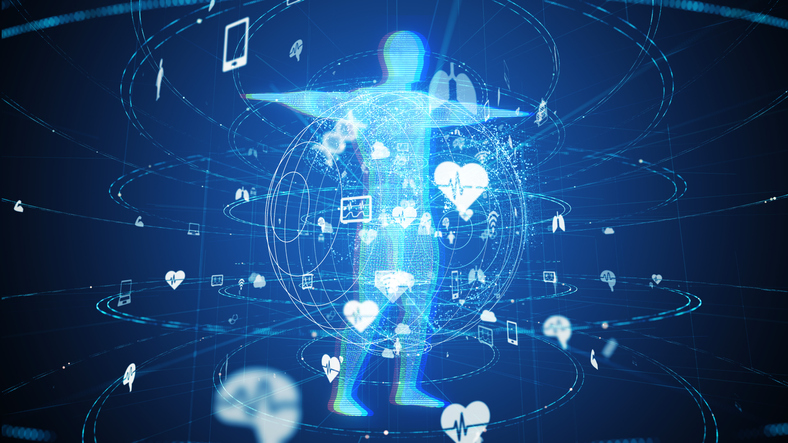
With the Rise of AI, What IP Disputes in Healthcare Are Likely to Emerge?
Munck Wilson Mandala Partner Greg Howison shared his perspective on some of the legal ramifications around AI, IP, connected devices and the data they generate, in response to emailed questions.
Gary Seamans, IDx CEO, made the case for the need for a failsafe because technology products inevitably will fail to do what they are supposed to do. He said if the product fails to make a diagnosis of diabetic retinopathy, the patient is required to be referred to an eye specialist. The rationale is the risk is too high that an abnormality exists and should be confirmed or refuted by a specialist with an in-person perspective.
The development of autonomous machine learning algorithms requires companies to consider technical, legal, and regulatory issues in the context of their products.
“Do you have a black box? How do you explain what you trained [ the computer] on? How do you explain how it works? As AI proliferates, that failsafe needs to be part of the product,” Seamans said.
Every AI tech is based on probability metrics, Arterys Head of Corporate Development Carla Leibowitz said. Speaking after the panel discussion, she clarified how her company addresses the challenge of when things go wrong with AI.
“We are always augmenting the radiologist. We automatically measure things that are hard to measure really well,” Leibowitz said. “If they can’t measure something, it will say ‘can’t measure it’, and the physician will do it manually. A lot of what we built into our system is this belt and suspenders approach — sometimes it will be great, sometimes physicians will want to tweak it and sometimes they are going to want to completely override it.”
Michelle Marlborough, AICure Chief Product Officer, noted after the session that the company’s applications are constructed to allow patients to manually override them if necessary. If AICure’s app doesn’t recognize a pill, patients can just override it and report they’ve taken their drug.
“We have two AI computer interfaces and one is for patients from the app point of view on their mobile phones and the other runs on the backend to detect fraud, cheating, the things that are flagged by the system and checked by humans.”
Correction: An earlier version of this article incorrectly referred to IDx’s technology as being used to detect diabetic neuropathy. It is used to detect diabetic retinopathy. We regret the error.