For many diseases, early diagnosis and intervention remain key to good outcomes. That has been the rationale behind screening tests for numerous diseases including colorectal cancer. But like many startups that are looking for clues in data, Medial EarlySign — based in Hod HaSharon, Israel — is leveraging artificial intelligence to mine EHR data to detect colorectal cancer risk much earlier.
Pushing diagnosis further upstream could have many benefits and not just for patients in terms of better outcomes. If the data reveals risk it can push people to take screening tests, that they otherwise don’t take. It also has the potential to save costs as the disease burden is lower early on. This is especially true of diseases like cancer, heart failure and diabetes. On Wednesday, Medial EarlySign launched two products intended to identify prediabetes patients as well as those Type 2 patients who are at risk of developing chronic kidney disease within three years. The AI tools will be featured at the annual meeting of the American Diabetes Association that kicks off in San Francisco next week
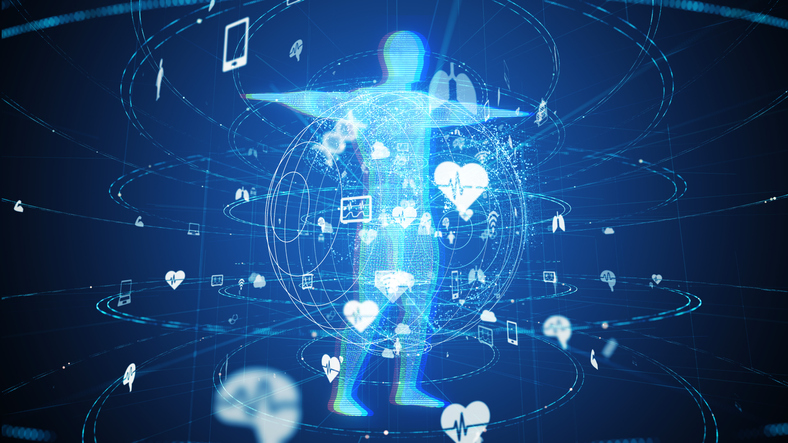
With the Rise of AI, What IP Disputes in Healthcare Are Likely to Emerge?
Munck Wilson Mandala Partner Greg Howison shared his perspective on some of the legal ramifications around AI, IP, connected devices and the data they generate, in response to emailed questions.
In Israel and the European Union, the company’s ColonFlag product produces a ColonScore to determine colorectal cancer risk in patients. In the U.S., on the other hand, a similar AI tool called LGI Flag aims to unearth lower GI disorders. Geva said that the product is FDA-exempt.
In January, SLUCare Physician Group, the academic medical practice of Saint Louis University, announced that it would begin to use LGI Flag on its patients to identify those at risk for lower GI disorders associated with chronic occult bleeding. These include polyps, irritable bowel disease, diverticulitis, ulcers, precancerous adenomas and colorectal cancer.
In an interview earlier this year, Medial EarlySign CEO Ori Geva said that the company’s machine learning algorithms have been trained on a multitude of EHR data. They have also been validated
“For example, the study we have done for lower GI has been validated by the University of Oxford on a population of 3 million individuals,” he said. “It has been studied and validated by Kaiser Permanente Northwest on a few hundred thousand individuals. And in all these cases, they contain hundreds to thousands of cases where the individuals were diagnosed, so …. there is statistical significance.”
Generally, once the patient is deemed at risk based on their EHR data, healthcare providers can recommend them to go for an actual diagnostic test.
In Israel, ColonFlag has been used for the past three years on patients between 50 and 75 who have not undergone either a colonoscopy or the Fecal Occult Blood Test (FOBT). In that period, the product was used for approximately 220,000 patients, of which, 1,913 patients were found to be at high risk of developing colorectal cancer, said Professor Varda Shalev, managing director of the Morris Kahn & Maccabi Research and Innovation Institute at Maccabi Healthcare Services, in an email. Maccabi Healthcare Services is the second-largest managed care organization in Israel, providing both care and insurance coverage for its members.
Once a high-risk score is found, the physician in care of that patient is alerted who generally will ask the patient to undergo a colonoscopy.
“In two years, we have found 67 patients with malignancy and 254 patients with polyps,” Shalev said. “There is a high probability that all of these would not have been discovered otherwise, since they had not performed screening checks.”
The benefit of the AI tool is that it is passive — in other words, patients are not being asked to do anything, he explained, and yet their proclivity for risk is gleaned just from the data. Shalev noted further that ColonScore could help to identify patients outside of the screening age parameters (50-75) and that is “highly desirable since the age of diagnosis is dropping significantly.”
For now, the company is relying on EHR data given that Medial EarlySign began on the provider side. But claims data may be used in training the AI as well. As such both payers and employers can become customers of the company, Geva said.
Medial EarlySign has raised $55 million in two rounds of funding for its HIPAA compliant tools and investors include Horizons Ventures that manages the private investment of Hong Kong business magnate and investor Li Ka Shing, and aMoon, a health tech and life sciences venture fund in Israel.
While Medial EarlySign is using data to predict risk that can trigger early intervention and reduce cost, a U.S.-based competitor is using AI to help payers identify which group of patients represent the highest cost burden in its member base, among other things. San Mateo-based Lumiata’s Health AI Cost Prediction can allow payers to better manage their overall health expenditure and more effectively allocate resources, while also optimizing the underwriting of large group renewals the payer may have with employers, according to its website.
However, just having a great predictive analytics tool doesn’t mean that the end-goal — be it better cost management or improved patient outcomes — is immediately achievable.
In a 2017 paper published in the Annals of Surgery, Alex Sox-Harris, a researcher with the Veterans Affairs Palo Alto Health Care System who won a Presidential Early Career Award for Scientists Researchers in 2009, explained that many things have to happen before a predictive surgical model can lead to better outcomes.
“In rare cases when a particular implementation of e-HPA (electronic health predictive analytics) is evaluated and shown to produce real improvements in treatment quality and outcomes, it is generally under-appreciated how many intervening steps happened to translate model predictions into improved health,” he wrote. “Before buying or using an e-HPA system, all stakeholders should insist on research that rigorously evaluates not only the accuracy of the predictive models, but their effects on health outcomes when used in particular ways in real clinical settings. Without evidence of effectiveness and a careful examination of unintended consequences, implementation of e-HPA systems may produce more risks and/or costs than benefits.”