Artificial intelligence (AI) is shaking up the healthcare industry. With applications in drug discovery, medical imaging, disease modeling and clinical trial conduct, it promises to revolutionize the ways in which we perform research, treat disease and work with patients.
In drug discovery, we have seen some of the realization behind the hype and early demonstrations of AI enabling target identification and pipeline development. AI can also support diagnostic decision-making in the medical imaging space, reading scans with exceptional speed and accuracy and detecting abnormalities invisible to the human eye.
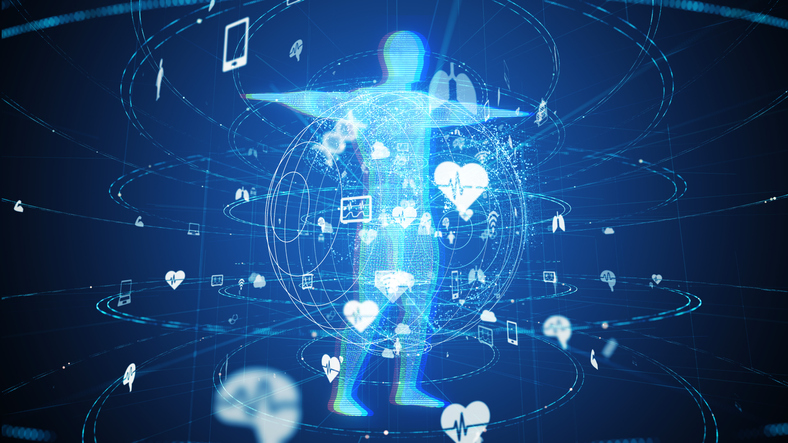
With the Rise of AI, What IP Disputes in Healthcare Are Likely to Emerge?
Munck Wilson Mandala Partner Greg Howison shared his perspective on some of the legal ramifications around AI, IP, connected devices and the data they generate, in response to emailed questions.
AI-enabled disease modeling, meanwhile, provides a more in-depth understanding of the etiology, transmission and progression of illnesses such as motor neuron disease, cancer and HIV. One of the most promising frontiers in this space, however, is the conduct of clinical trials and enhancing the probability of regulatory or technical success.
Increasing a clinical trial’s chance of success requires the careful alignment of several different factors, with clinical trial sponsors looking for solutions which minimize timelines whilst maximizing outcomes. There are various operational and scientific decisions to be made in the clinical trial process—from site selection to endpoint selection—that can help to de-risk trials and lead to more successful outcomes. Increasingly, AI is being used to help study teams solve some of the challenges they face—whether operational, scientific or ethical.
AI produces actionable operational insights
From an operational standpoint, trial sites can vary in terms of their performance, particularly with regards to the speed and diversity of patient enrollment. Through AI analysis, sponsors and contract research organizations (CROs) can leverage historical trial data or real world data to better understand site performance, and thus make more informed decisions with regards to time and resource allocation.
This knowledge and oversight can result in shortened development timelines, which ultimately benefits patients. This use of AI has been particularly important in the face of Covid-19, where AI has proven invaluable in rare disease and oncology trials by helping sponsors make rapid pivots based on real-time predictions and insights based on backlogs at trial sites due to an influx of Covid patients. While still in its early days, AI is being used to assess data on patient availability and diversity, thus enabling sponsors and CROs to de-risk their decisions in a competitive landscape.
Scientific hypotheses can be pressure tested by AI
The recipe for trial success requires deep understanding of the disease in question, the patient population it affects and the potential treatments. Historically, this has been achieved through review of scientific literature and preceding clinical research.
AI is now being used to augment the intelligence underpinning a trial. By analyzing multiple sets of inputs, including historical trial designs, drug biology, sponsor characteristics and clinical trial outcomes across development programs, it allows us to sharpen protocols and accurately predict trial success.
In particular, incorporating real world data alongside clinical trial data can provide deeper clinical insight into patient outcomes and improve risk monitoring. It can also support decisions around endpoint selection, better equipping sponsors and CROs to target the best and most clinically relevant endpoints possible. AI is also being used to flag real-time trends emerging in trials that might otherwise not have been obvious until the end of a study when all the data is analyzed.
AI supporting more diverse trials
One further challenge that has long plagued clinical trials is a lack of diversity of trial participants. From both a scientific and ethical standpoint, it is essential to address the underrepresentation of certain populations within trials. Research that fails to address different ethnicities, ages, genders and lifestyles will not result in impactful treatments that are representative of patient populations.
AI can play a role in bridging this gap, through identifying which trial sites are best placed to serve underrepresented communities. By simulating patient models, certain conclusions and hypotheses can be reached about the proportion of patients in a subgroup who will respond to a particular treatment. This can inform how clinical trial teams think about recruitment and the diversity of recruitment. However, those involved in developing and employing AI systems need to pay close attention to dismantling rather than reproducing bias in their collection and use of data. This includes building models which are translatable to a broad, epidemiologically representative population. As ever, regulation has a role to play in shaping approaches to risk management, data provenance and mandating transparency.
Synthetic control arms as a powerful data-enabled tool
Synthetic control arms (SCAs), also known as external control arms, are another innovative tool enabled by big data, powerful computing and advanced analytics. While AI serves to mimic real life, SCAs use actual, patient-level data and biostatistical methods to replicate a control arm, removing the need for a placebo group.
Similarly to AI, these advanced statistical methods and analytics require huge amounts of data to accurately emulate real life. While well-established biostatistical approaches may fall outside of the definition of “AI,” it’s important to note that traditional methods paired with high quality data have shown great promise and success in regulatory settings.
Beyond diversity, patient recruitment comes with other challenges, particularly the time pressure to recruit as quickly as possible, as well as the ethical implications of recruiting for a control arm of a trial for conditions where there may not be effective treatments available, such as many rare diseases. Synthetic control arms create a proxy for real clinical trial patient-level data and can offer representative datasets that provide valuable information about a disease, indication or treatment.
Additionally, models can be run iteratively, meaning that dynamic datasets can be run through a variety of analyses to model for several different outcomes. A small number of synthetic control arm submissions have been approved by the FDA, including one for a hybrid design in a phase III trial in recurrent glioblastoma, an illness with few treatment options and high unmet need. SCAs are just one of a myriad of advanced analytical tools and statistical methods with huge potential in the clinical stages of drug development.
The untapped potential of AI in clinical research
By tapping into the power of AI, we have gained a deeper understanding of disease, patient populations and potential treatments. Technology is transforming the way we run clinical trials: It is improving elements of trial design, including target population selection, comparator arms and clinical endpoints. It is also improving patient safety and patient enrollment and giving pharmaceutical companies crucial insights and analysis into how their drugs work. But we’ve only just scratched the surface of what we can truly achieve. The potential is enormous and AI is certain to become an essential part of clinical research and drug development in the future.
Photo: Blue Planet Studio, Getty Images
Arnaub Chatterjee is Senior Vice President of Acorn AI, a Medidata company. Medidata is the global market leader in clinical data management in life sciences and in his role, Arnaub leads the development of data science products to advance research, development and commercialization. In addition to his role at Medidata, he also serves as Teaching Associate in the Department of Health Care Policy at Harvard Medical School and Lecturer in the Department of Policy Analysis and Management at Cornell University.
Prior to Medidata, Arnaub was Associate Partner in the Pharmaceutical and Medical Products group at McKinsey & Company, and Director of Merck's Data Science and Insights group. He served in the Obama Administration as an advisor to former Chief Technology Officers at the U.S. Department of Health and Human Services (HHS). His work has been published in journals such as the Harvard Business Review and Nature.