Coined in 1956, the term “artificial intelligence” (AI) has become a popular phrase today to describe a variety of benefits across multiple industries – from performing frequent, high-volume, computerized tasks to adding a layer of “intelligence” to existing products. AI was created to mimic human intelligence, and – since its implementation – the process has been refined, producing more specific subsets of AI, including machine learning and deep learning. These applications are beginning to play a large role in a variety of fields, including healthcare. Since its inception, AI has revolutionized every facet of medicine – from alleviating the manpower needed for administrative tasks in hospitals and clinics to providing huge advancements in the medical device space. However, the areas that will undoubtedly benefit the most from AI are big pharma and biotechnology.
Its important role in advancing medicine
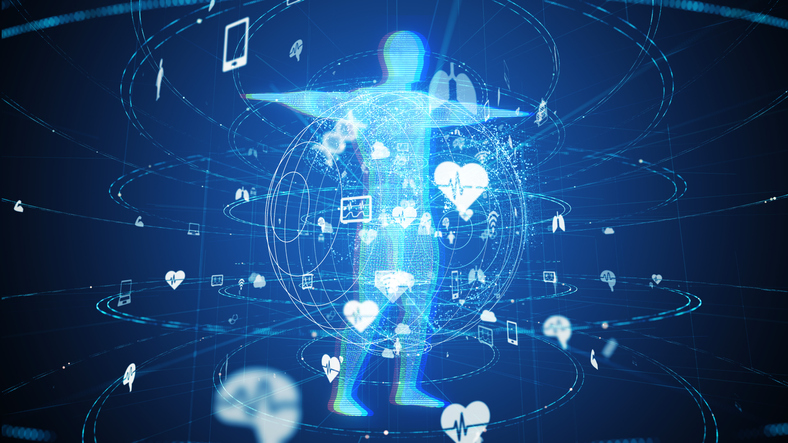
With the Rise of AI, What IP Disputes in Healthcare Are Likely to Emerge?
Munck Wilson Mandala Partner Greg Howison shared his perspective on some of the legal ramifications around AI, IP, connected devices and the data they generate, in response to emailed questions.
Biotech companies are using AI and machine learning to expedite the drug discovery process, with the potential to create dozens of new medicines in the next decade. Traditionally, much of the drug discovery process has been expensive, time-consuming guesswork, with experiments taking months or even years to produce outcomes. The implementation of AI is significantly cutting that timeline down by combing through vast data sets to quickly identify patient response markers and create viable drug targets more cheaply and efficiently. According to Morgan Stanley Research, “Modest improvements in early-stage drug development success rates enabled by the use of artificial intelligence and machine learning could lead to an additional 50 novel therapies over a 10-year period, which could translate to a more than $50 billion opportunity.”
According to a GlobalData survey, 39 percent of surveyed healthcare industry professionals believe AI will trend as the most disruptive emerging technology in the sector in 2023. Despite a variety of factors impacting the pharmaceutical industry over the last few years – from the Covid-19 pandemic to supply chain issues and subsequent inflation – partnerships between pharma companies and AI-tech providers have continued to grow, especially in the field of R&D. The same report also suggested that Big Data was thought to be the most important partner to AI, in terms of introducing technology-related changes in the pharmaceutical sector.
Big Data and the drug discovery process
A Journal of the American Medical Association (JAMA) report from 2020 concluded that the average cost estimates to bring a drug to market ranged from $765.9 million to $2771.6 million, depending on the specific therapeutic area. One important way big data assists in drug discovery is through predictive analytics, which is the machine learning process of using data to make predictions about future outcomes. Pharmaceutical companies use these complex algorithms in addition to large amounts of raw data to generate predictive models. This means researchers can predict how drugs will interact, their toxicity, and any potential inhibitions, saving a significant amount of time. Big data also serves to amplify drug discovery with software algorithms that correlate with drug data, indicating whether a drug needs additional fine-tuning or predicting a drug’s potency. By allowing researchers to leverage factors such as genetic makeup, disease status, historic patient data, demographics, past clinical trial data, and more, big data saves both time and money while simultaneously maximizing a study’s success rate.
AI’s ability to improve cancer detection and treatment
With imaging tests, doctors can detect cancer early, determine the stage of tumors, and monitor the effectiveness of treatments. In the last several years, researchers have developed AI tools that have the potential to make cancer imaging faster, more accurate, and even more informative. AI has the potential to identify complex patterns and relationships between very different kinds of data, often times “seeing” correlations that humans cannot. For example, one group of scientists created an AI algorithm to help determine how often someone should get screened for breast cancer. By using an individual’s mammogram images, the model could predict that individual’s risk for developing breast cancer over the next five years. After multiple tests, the model was more accurate than the current tools used to predict breast cancer risk. Although the potential for AI to aid in the detection and treatment of various cancers is promising, there is still much work to be done to ensure these applications are ready for real-world use.
How it accelerates antibody drug discovery
AI and deep learning continue to impact complex tasks, such as design and optimization of antibodies. In 2021, five out of the top 10 selling drugs were monoclonal antibodies (mAbs), and this trend is forecasted to remain the same over the next five years. Monoclonal antibodies are delivered to the body intravenously in an effort to change a person’s genetic makeup and help them more effectively fight diseases. With the global monoclonal antibodies market predicted to hit around $500 billion by 2030, the mAbs market is making waves across big pharma and shows no signs of slowing down in the near future. While there are multiple methods to generate fully human antibodies, the trends have been changing towards isolating antibodies directly from humans. Techniques such as next generation sequencing, high-throughput robotic screening, B cell sorting, and single cell sequencing are expected to greatly accelerate the identification of specific binders, facilitating mAb development for use in research, clinical trials, and pharmaceuticals for the treatment of a variety of human diseases.
Some companies are also using models in order to identify a template antibody against a desired target from existing human antibodies, then using a different machine learning model to predict mutations and guide evolution of the identified template to improve affinity – the strength by which two (or more) molecules interact or bind – and other biophysical properties.
Traditionally, pharmaceutical companies have been slow to adopt these newer technologies, instead relying on well-established and proven – but usually complex – manufacturing processes. However, the time to begin investing in – and exploring – AI, machine learning, and big data is now. Companies who do not embrace these new technologies are sure to fall behind, as AI will only continue to revolutionize the drug discovery game.
Photo: metamorworks, Getty Images
Dr. Chan is a physician-inventor. He is currently Chairman, Co-Founder of Abpro, CEO of rHEALTH, and President, CSO of DNA Medicine Institute, a medical innovation laboratory. He has been honored as Esquire magazine’s Best and Brightest, one of MIT Technology Review’s Top 100 Innovators, and an XPRIZE winner. His work has contributed to the birth of next-generation sequencing, health monitoring in remote environments, and therapeutics. Dr. Chan holds over 60 patents and publications, with work funded by the NIH, NASA, and USAF. Dr. Chan received an A.B. in Biochemical Sciences from Harvard College summa cum laude in 1996, received an M.D. from Harvard Medical School with honors in 2007, and trained in medicine at the Brigham and Women’s Hospital.