Healthcare organization CFOs are facing a perfect storm of challenges around medical coding.
With few workers entering a field dominated by older Gen X-ers and Baby Boomers, there’s a growing gap in skilled medical coders — people who read notes from doctors and apply appropriate codes for billing and reimbursement purposes. Understaffed medical coding teams are struggling to keep up with demand. And physicians — who should be treating patients, not coding — are also feeling the burden of having to correct coding errors.
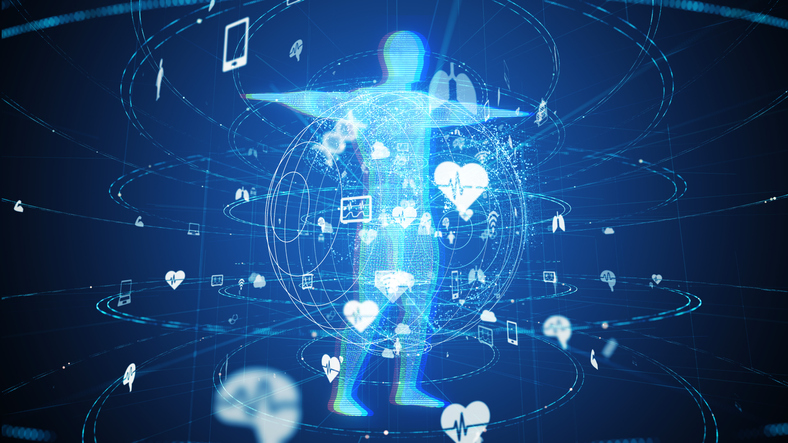
With the Rise of AI, What IP Disputes in Healthcare Are Likely to Emerge?
Munck Wilson Mandala Partner Greg Howison shared his perspective on some of the legal ramifications around AI, IP, connected devices and the data they generate, in response to emailed questions.
Some health systems are offshoring coding work, but that comes with its own set of concerns and quality issues. With a finite number of staffing resources to put towards the growing manual work, health systems are seeing higher rates of coding errors, which means more denials and billing delays.
For CFOs looking to solve these challenges in a way that both improves the bottom line and supports their people, a better path forward is to automate parts of the coding function.
Artificial intelligence (AI) makes coding more efficient and accurate, and streamlines the revenue cycle. This eases the workload for medical coders and relieves doctors of unnecessary administrative burden. It also helps CFOs to cut costs, avoid lost revenue, and significantly reduce denials.
The value is there, but so is the skepticism. And understandably. CFOs have been burned in the past by AI technology “improvements” that promise the world but fail to scale and adapt, or that require ongoing resources to keep pace with ICD and CPT updates. Before you take the leap, here’s what you need to know about AI-powered medical coding and how to set yourself up for success.
Medical coding requires AI that adapts
One of the most important things to consider when looking to use AI is adaptability. Does the AI system make decisions based on manual input? Or is it driven by data? Do you have to adapt to the AI system? Or does the AI system adapt to you?
Many AI solutions use Natural Language Processing (NLP) and Natural Language Understanding (NLU) to extract and synthesize information from doctor’s notes. For example, NLP & NLU can be used to determine that ‘pleural effusion’ is a medically interesting phrase. But from there, AI solutions differ in how they take those medically interesting words and phrases that appear in a document and make that information actionable.
Traditional rules-based systems require teams of humans (including medical coders, residents, nurses, and/or doctors) to create a rule. For example, a rule may be: when the words ‘pleural effusion’ appear together, in specific circumstances, code it a certain way. Every time there’s a change, whether it’s updating ICD and CPT codes, changing your documentation style, or bringing on a new physician practice, humans must often rewrite the rules.
In comparison, a machine learning system autonomously learns generalizable rules to translate medical phrases from doctors’ notes into codes. As coding gets more complicated with new and changing ICDs and insurance requirements, having a system that adapts to your documentation style, workflows, and processes means that you don’t have to constantly be in building and rebuilding mode. It’s the difference between a robust system or a brittle system that breaks with every update.
Want hands-off coding? Incorporate quality checks
Fully autonomous coding means that an AI platform can read the doctor’s notes, decide the appropriate codes, assign those codes to the case, and send it directly to billing. This automation is already possible today.
However, touchless coding isn’t something you apply across the board. A case must pass a series of quality checks to account for the complexities of revenue cycle management. Start with these three questions:
- Do the AI-derived codes pass prediction confidence thresholds to meet health system-specific quality targets?
It’s important to know what quality you need across different cases. When AI clinically codes a case and you have confidence that it’s meeting a quality target, then you can consider the code automated. For example, a healthcare organization may require a 98% quality target for MRIs and CTs, but only a 92% quality target for chest x-rays. These targets are established based on medical specialties (radiology, surgery, and so forth) and where the health system believes the codes will not result in a denial and/or will satisfy other quality needs.
There’s a tradeoff here: the higher quality you demand in a certain area, the less you’ll be able to automate.
- Do the predicted codes pass payer-specific rules?
Medical coding requirements can change by payer and state. Take, for example, a patient who has conscious sedation during a colonoscopy. You may need to code that one way for Medicare and another way for a private insurer. The same goes for workers’ compensation coding and billing requirements, which vary state to state.
To ensure accurate and timely reimbursement, it’s critical that each case is assigned the correct billing code. That way, the ICD codes on the medical side align with the CPT codes on the payer side to ensure quick payment. If codes are incorrect, up-coded, or down-coded, it introduces churn to the revenue cycle as cases are denied and sent back through the system for corrections.
- Are there any issues that would cause this claim to be denied?
Using a claims scrubber to review medical claims before they’re submitted to payers must become a standard part of your revenue cycle management if it isn’t already. It’s a crucial quality check within an autonomous process that acts as the last chance to correct any errant codes.
If a case fails to pass any one of these rev cycle quality checks, it needs to be reviewed by a human and coded manually. Only when a case passes them all can it be sent straight to billing and no human being has to touch it.
The goal isn’t to automate every single case. Rather, health systems are looking to AI and automated workflows to handle simple coding tasks, such as examinations and imaging requests—though as the systems become more sophisticated, they will inevitably learn to handle more complex tasks. In doing so today, some health systems are able to automate 75-85% of all radiology cases. That way, professionally trained medical coders can focus on more complex specialties — like interventional radiology (IR) and complex surgical cases — that need a human touch.
Every healthcare organization has different opportunities for AI
When incorporating AI in medical coding, every health system has different specialties where it can make the most immediate impact.
For one, it may be getting doctors out of the business of coding. AI can free up physicians from having to spend time making coding adjustments so they can get back to what they are meant to be doing — treating patients. For another health system, it may be relieving the pressure valve on overwhelmed coding teams, who can’t keep up with demand.
Chances are, you’re trying to save money and not waste resources — but how? Are denials through the roof? Are you under-staffed? Are you facing attrition? Is there a department or specialty area that is more prone to errors?
Answering these questions will help you figure out where there’s the greatest need and where to start so you can set expectations and lay the foundation to map the impact of AI on bigger-picture financial and business goals.
An automated future that’s better for your people
We’re not looking at a future of medical coding with zero human intervention. We’re looking at a future that makes employees’ work lives better — from coders to physicians. And in the process, health systems ensure accurate coding and quicker billing that lead to fewer denials and higher cost savings that ripple across the organization.
Photo: Andrzej Wojcicki, Getty Images
Dr. Javed (Jay) Aslam is the Chief Data Science Officer at CodaMetrix. In his role as CDSO, Jay oversees all Artificial Intelligence, Machine Learning, and Natural Language Processing at the company. He was part of the team that developed Massachusetts General Brigham’s original medical coding AI system in 2016. Jay is also a Professor at Northeastern University where he most recently served as the Senior Associate Dean for Academic Affairs in the Khoury College of Computer Sciences. Prior to joining Northeastern University, he was an Assistant Professor in the Department of Computer Science at Dartmouth College, and he held a postdoctoral position in the School of Engineering and Applied Sciences at Harvard University. Jay received his Ph.D. in Computer Science from MIT, with a focus on machine learning and noise-tolerant computation.