While leaders across the healthcare industry are certainly excited about generative AI and its potential to alleviate clinical and operational inefficiencies, they are also carefully considering how to mitigate risk associated with the use of these novel tools. The stakes are high in healthcare — one slip-up could be the difference between life and death.
The rise of generative AI in healthcare is still pretty nascent, so the industry is still figuring out how to create guidelines that ensure these AI models are deployed responsibly. For example, more than 30 health systems and payers from across the country came together last week to launch a collective called VALID AI, which aims to explore use cases, risks and best practices for generative AI in healthcare and research, with hopes to accelerate ethical adoption of the technology across the sector.
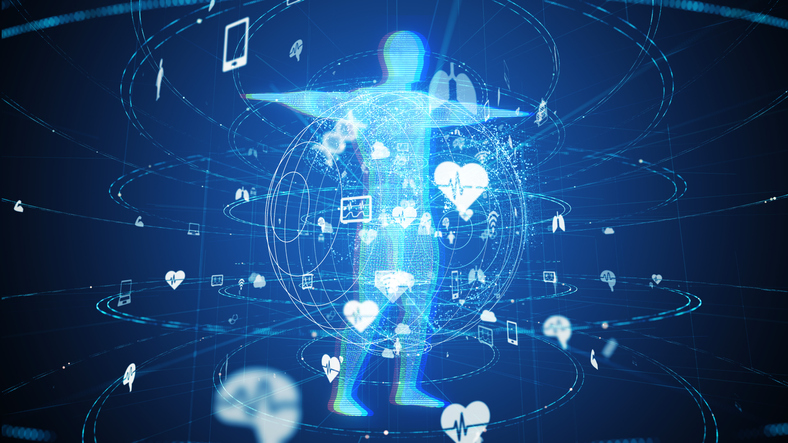
With the Rise of AI, What IP Disputes in Healthcare Are Likely to Emerge?
Munck Wilson Mandala Partner Greg Howison shared his perspective on some of the legal ramifications around AI, IP, connected devices and the data they generate, in response to emailed questions.
John Halamka, president of Mayo Clinic Platform, gave a window into how his health system is approaching this issue last week during an interview at HLTH in Las Vegas.
One thing healthcare professionals need to keep in mind is that all AI models are biased, he pointed out.
“They just are. You may do your very best to train it on the most extraordinary data. But there are always going to be patients that aren’t in EHRs, or aren’t represented in a training set because they’re in a rural area, or maybe they fear going to clinicians,” Halamka explained.
At Mayo Clinic, his team works hard to identify these kinds of biases and mitigate them, he said.
If Mayo Clinic develops a new algorithm, its researchers will run subgroup analyses for the model, Halamka explained. For example, they will run analyses for older versus younger populations, white versus Black populations, urban versus rural populations, male versus female populations, or high-income versus low-income populations.
If the algorithm doesn’t work as well for a certain group, Mayo Clinic “puts a warning label” on the model so that it’s not used for that population. Then, they get to working on building a model that can better serve that group.
This type of nimble approach is key when it comes to generative AI, Halamka noted. It seems like AI is getting more advanced each week, so healthcare leaders need to get speedier with their bias mitigation strategies, he said. This is especially important because “every stereotype in any literature is going to be pixelated into generative AI,” Halamka pointed out.
To illustrate this point, Halamka asked me to type “women cannot _____” into ChatGPT. He warned me that it would say things like “women cannot drive” and “women cannot be president,” as that is what the chatbot told him just a couple weeks ago. However, when I followed Halamka’s instructions, ChatGPT produced a paragraph letting me know that it’s inappropriate to make blanket statements about what women can or cannot do. OpenAI had already refined its model.
Given that OpenAI is still working on fine tuning its generative AI model and ridding it of biases, AI developers in healthcare need to be doing the same and watching out for these kinds of problems, Halamka said.
“It’s a really hard thing to take the bias out of generative AI. One of the things that Mayo will do is it will train foundational models from scratch using only curated clinical information from Mayo Clinic that has been deidentified, with the hope that we’re reducing some of the stereotypes and mythology and memes that you could get out when generative AI is trained on Reddit,” he declared.
Generative AI is only as good as the data it’s trained on, Halamka noted.
Photo: Natali_Mis, Getty Images