The numbers in drug discovery are daunting. Identifying a molecule with therapeutic promise and advancing it through preclinical and clinical development can take more than a decade at costs topping $2 billion, according to pharmaceutical industry observers. Meanwhile, drugs fail in clinical trials more than 90% of the time.
Artificial intelligence in particular is being touted as a savior against the pitfalls of discovering and developing new drugs. Several companies have staked claims about being first or faster with their AI platforms, but none have yet taken a fully AI-generated molecule from discovery all the way through clinical development. And despite the widespread portrayal of AI as game-changing in all aspects of healthcare, in drug research, the wait could be a little longer.
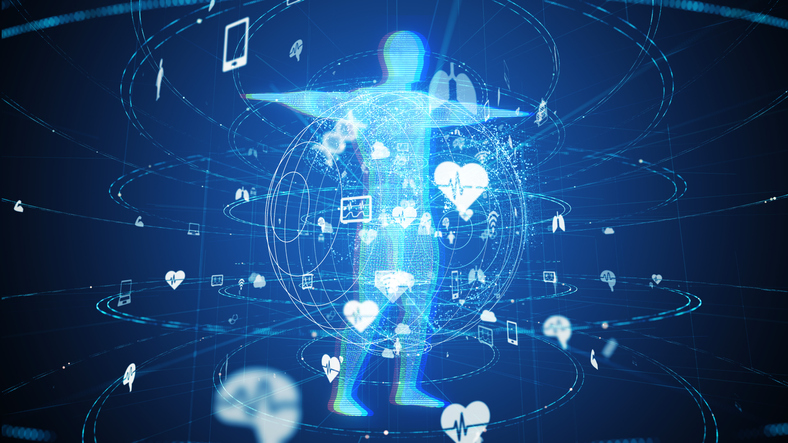
With the Rise of AI, What IP Disputes in Healthcare Are Likely to Emerge?
Munck Wilson Mandala Partner Greg Howison shared his perspective on some of the legal ramifications around AI, IP, connected devices and the data they generate, in response to emailed questions.
While AI has helped companies more quickly identify drug targets and discover new molecules to hit them, some of the clinical trial results have been disappointing. In the past year, AI-discovered molecules have fallen short in clinical trials in atopic dermatitis, schizophrenia, and cancer. Consequently, companies have shaken up pipelines and investors have taken pause.
Individual efforts may have faltered, but that doesn’t mean AI has failed, said Andrew Hedin, a partner at Bessemer Venture Partners who co-leads the firm’s healthcare practice.
“Pipeline prioritization and clinical trial risk exist for any biotech, regardless of their technology,” Hedin said. “It’s always been unrealistic to think that AI is a cure-all that will lead to 100% success rates in drug discovery and it’s also too simplistic to lump every AI-enabled drug together. There’s a breadth of AI applications with rapidly improving models and data sets to unlock scientific progress that will undoubtedly have a positive impact on patients’ lives.”
Continuous Learning With AI
In some ways, AI is not new. Computational techniques have long been applied to drug research. What’s changing is the data that are analyzed and the technologies used to interrogate them. Exscientia is among the AI-focused companies whose approach employs generative AI, which is the generation and analysis of far more data than is possible with traditional drug discovery methods. When the Oxford, United Kingdom-based company went public two years ago, its most advanced internally developed program was EZXS21546, an immuno-oncology drug in early clinical development in kidney and lung cancers. In early October, the company announced the wind-down of that program.
Exscientia concluded it would be challenging for its drug to achieve a suitable therapeutic index, which is the dose range that balances safety and efficacy. The company also said its decision was supported by recent peer data. In August, Gilead Sciences-partnered Arcus Biosciences said it would deprioritize its prostate cancer drug candidate, which goes after the same target as Exscientia’s former lead program. Arcus said an analysis found its molecule was not expected to achieve enough clinical benefit.
Ben Taylor, Exscientia’s chief financial officer and chief strategy officer, said the discontinuation decision followed a review of published data on related molecules as well as data the company generated internally. Taylor distinguishes Exscientia from others in drug discovery by describing it as “a learning company,” which refers to the overall process of learning rather than specifically using machine learning technologies. Exscientia focuses on answering specific questions about drug design and precision medicine where little data are available and screening would not be feasible, he said. Generating data and feeding data into the platform enable Exscienta’s scientists to continuously learn. This process informed the decision on the cancer program.
“We believe in making data-driven decisions at the earliest possible time point, so we made the strategic decision to discontinue ’546 and divert the resources to some of our other highly differentiated programs that have a better risk profile,” Taylor said.
Exscientia does not describe its cancer program’s outcome as a failure—the trial did not read out data. But the outcomes for AI-discovered drug candidates from BenevolentAI and Sumitomo Pharma were clearly clinical trial failures.
BenevolentAI’s former lead drug candidate blocked three enzymes that have already been addressed by cancer drugs. The company’s technology identified these enzymes as having an additional role in itch and inflammation. However, its molecule, developed as a treatment for atopic dermatitis, posted Phase 2 results in April showing it failed to achieve the study’s itch and inflammation reduction goals. Soon after, the company announced a corporate restructuring and a shift in pipeline priorities that ended the atopic dermatitis program.
The clinical trial failure of Sumitomo Pharma’s AI-discovered asset was financially more costly than BenevolenAI’s, coming in two Phase 3 studies. Developed as a treatment for schizophrenia, the Japanese drugmaker identified its molecule through a partnership with PsychoGenics, a company whose technology helps its pharma industry partners discover drugs for central nervous system and psychiatric disorders.
According to PsychoGenics CEO Emer Leahy, the best way to learn how an experimental molecule affects behavior is to administer it to a mouse and observe behavioral changes. Cameras aimed at the mice employ a type of AI technology called computer vision to collect millions of data points. Data analysis defines behaviors and behavioral patterns in the mice, which in turn enables scientists to select the molecules with the best potential to achieve the desired behavioral effect, she said.
The PsychoGenics research identified a molecule that Sumitomo advanced into clinical trials. However, the statistically significant improvement detailed in published data from a placebo-controlled Phase 2 study was not replicated in the larger Phase 3 tests. Sumitomo attributed these results to a high placebo response.
In Sumitomo’s report of fiscal second quarter 2023 financial results in October, the company said analysis of the failed Phase 3 studies is ongoing. Goals include trying to understand the large placebo effect. For Sumitomo, there’s more at stake than schizophrenia. Its AI-discovered molecule is in Phase 2 development in Parkinson’s disease psychosis and in Phase 3 testing in generalized anxiety disorder and major depressive disorder.
A Fast Path to “Target X,” But Few Details
The disappointing readout of Sumitomo’s schizophrenia trial data came as Insilico Medicine boasted of having the first AI-discovered drug reach mid-stage clinical development. The Hong Kong-based company uses proprietary AI technology to discover targets and design molecules with desirable drug properties. To date, Insilico says its technology has yielded 31 programs addressing 29 targets. Most are in preclinical development for cancers. But most of the attention on Insilico has focused on its idiopathic pulmonary fibrosis (IPF) program, which started Phase 2 testing this year.
Insilico’s drug is intended to be an alternative to the two older FDA-approved IPF therapies that have limited efficacy and a long list of side effects. While Insilico is not the only company developing a new IPF drug, unlike the others it has not disclosed the target of its research.
Insilico claimed it was able to go from target hypothesis to preclinical validation of its IPF drug candidate in 18 months at a total cost of $1.8 million—a fraction of the time and cost typical of traditional drug discovery. However, while the company has published research about its cancer programs and their targets, there are no published papers about its IPF work. The company describes the target of its small molecule for IPF only as “Target X.”
The identity of Insilico’s IPF target remains undisclosed for competitive reasons, said Brita Belli, Insilico’s head of public relations. But she added that the company can say the target regulates at least three pathways implicated in fibrosis: YAP/TAZ, Wnt, and TGF-beta. That description suggests Insilico’s IPF approach overlaps with some other IPF drug candidates in development. Insilico expects data from the placebo-controlled Phase 2 trial of its IPF molecule will become available in 2025.
Hussein Jaafar, a consultant at Lifescience Dynamics, a London-based biopharma industry consulting firm, says companies are turning to AI because the low-hanging fruit of easy drug targets has already been picked. The early stumbles of some AI-discovered drug candidates do not worry him. The industry as a whole already has a high failure rate—AI won’t make that go away. The important question is whether AI can improve the failure rate, even a little, Jaafar said. Considering that a successful drug could bring in hundreds of millions to billions of dollars in revenue each year, even a modest increase in the success rate can reap big financial rewards. But judging the success of AI will take more time.
“We need to have dozens, if not hundreds of AI-enabled assets go through the clinical trial phase before we can justifiably say, yes the AI did help or not,” Jafaar said.
He added that the goal post measures of success for AI have shifted over time. AI has already been successfully used to find new targets and for improving clinical trial design, he explained. Technologies are now accomplishing things many in the industry did not think was possible a decade ago. For example, despite adoption of AI in target discovery, the synthesis of new molecules has remained manual—humans working in a lab. In recent years, AI and robotic technologies have been incorporated into this part of drug research. Jaafar said he believes a fully AI-generated drug will eventually reach the market. At that point, the question will shift from whether such a drug can get approved to how many can get approved per year, he said.
AI Biotech Startups Become Bilingual
Interest in AI-based drug discovery is evident in the investment dollars pouring into startups. But the industry may have already reached an investment peak. U.S. venture investment in AI biotech companies climbed steadily from 2015 until 2021, when $3.8 billion was spread across 108 deals, according to data compiled by PitchBook. In 2022, investment in AI biotechs dipped to $2.9 billion invested in 103 deals. Uncertainty in the financial markets is making fundraising difficult and the downward investment trend is continuing. As of mid-November, PitchBook counted $1 billion invested in 51 AI startups so far this year.
Hedin, of Bessemer Venture Partners, said startups have included AI in their pitches to his firm for the past decade, but the mentions of AI have proliferated in the last few years. One trend he sees is companies pairing traditional drug discovery scientists with experts from the tech sector, resulting in what he describes as “a bilingual team.” Some of these companies are going beyond using AI to make predictions. Now they’re generating new data, such as a novel molecular structure that would not have come from traditional molecular screening techniques.
“The predictions are obviously a function of how good the data is,” Hedin said. “Data in biology is often messy and not in a codified way that is amenable to traditional AI or [machine learning] approaches. That is one of the things that industry is working toward, creating a data set that is more usable for AI techniques.”
Iambic Therapeutics (not a Bessemer portfolio company) is one startup that fits the bilingual trend Hedin sees. The La Jolla, California-based company’s employee roster is evenly split between software engineers and drug discovery scientists, which co-founder and CEO Tom Miller said reflects the expertise needed for technology that employs AI to better understand known but elusive cancer targets. With the AI-derived insights, Iambic designs molecules able to bind to these targets while also offering better safety and efficacy, he said.
In October, Iambic unveiled $100 million in financing to support its plans to reach the clinic. Like many AI biotech startups, Iambic touts the speed of its platform. In the span of four years, Miller said Iambic produced four AI-discovered small molecules that the company believes have a higher chance of clinical trial success. He added that Iambic has never had to discontinue any programs due to a failure. But Miller, who is new to biotech after spending 14 years as a Caltech chemistry professor, tempers his optimism with a dose of realism. There’s a hazard in being overconfident that what’s observed in preclinical research will translate to humans, he said. Also, no matter how good any technology is, it’s never a shortcut.
“There are no shortcuts in drug discovery and development,” Miller said. “But there’s huge opportunity for technology to improve the success and to augment the ability of the people who are so important for delivering those drugs.”
Image: Getty Images