Radiology is an information technology business where data is the currency. Our modalities collect and process data; we analyze those visual and numerical data and convert them to auditory and written (“results”), which we communicate to patients and referring physicians. Technology has, heretofore, been our strong ally.
Our picture archiving and communication systems (PACS), reporting, and worklists are tools that have yielded significant improvements in productivity and communication with peers and patients. We can, however, do better––if we have better tools. It is commonly speculated that radiologists can eventually be replaced by AI, but I contend that by embracing innovative technology, we can be a model for similar enhancements used by our partners in care.
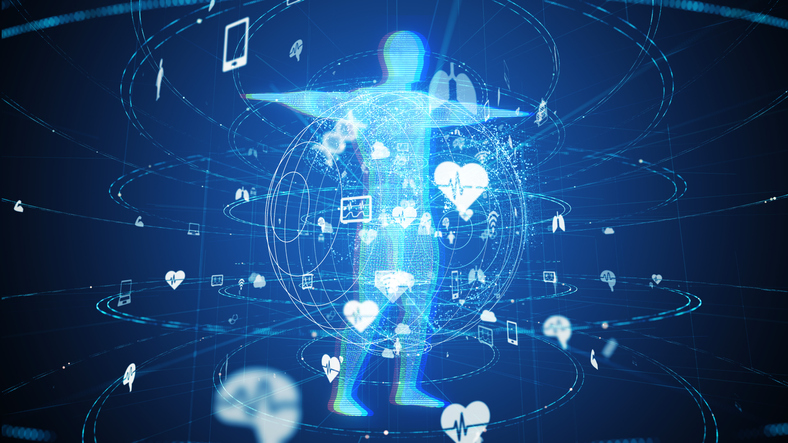
With the Rise of AI, What IP Disputes in Healthcare Are Likely to Emerge?
Munck Wilson Mandala Partner Greg Howison shared his perspective on some of the legal ramifications around AI, IP, connected devices and the data they generate, in response to emailed questions.
Radiology is at a tipping point, and which way it tips will be most influenced by the choices of radiologists. Will we take the path of least resistance and adopt sequential applications where machines assemble, collate and interpret the data from scanners? Or will we merge modern hardware and software to facilitate a new workflow for ourselves and our partners, thereby enhancing and cementing radiology’s central role in the care continuum?
Over the past decade, a concept of the evolution of radiology has been embodied by a term popularized by former Chair and President of the ACR Bibb Allen: Imaging 3.0. The three phases parallel the technological evolution of radiology from film to digital to advanced application of technology to provide value to patients. Radiology finds itself on the precipice of another technological catapult. Cloud-based availability and acceleration in computing power will enhance radiology’s centrality to patient care, allowing all parties involved––including the patient––to input and access vital data in more unified and meaningful ways. We can start with a redesign of our IT architecture and radiologist workflow (hub, nidus), which will lead the rest of the disaggregated data sources to a unified source of knowledge.
This will expand the 3.0 concept of “value” to what I call “Enterprise Knowledge.” Instead of stewards of aggregated data, radiologists can become creators of Knowledge that feeds into a patient’s electronic health record and informs the entire continuum of healthcare.
Let us see what the future of radiology might look like through this lens, applying a unified tech stack, artificial intelligence (AI), and a holistic approach to patient care.
Unified technology for powerful applications
Unification––stacking all the components into a single workflow––means there is real-time communication during the worklist construction and dissemination and the viewing-reporting process. A unified radiology stack is a more desirable, effective partner with GAI and AI diagnostic applications, bidirectionally leveraging the information contained in each to their mutually enhanced value. To the extent that information flows multi-directionally among the medical team, billing department, electronic medical record, etc., the interaction among all components improves the function of each.
For example, during the interpretive process, viewer and reporter are merged, so the dictation device and radiologist’s voice are no longer limited to controlling the construction of the report. Radiologists will keep their eyes on the images as voice commands control all the functions of the viewer, e.g., measurement, windowing, hanging protocols, etc. Specified images become a hyperlink in the report, so everyone has instant access without having to search. Imagine the benefits to the efficiency and understanding of the referring physician and patient––telemedicine consults, emergency room triage, surgeries, pharmaceutical research, and rehab, to name a few examples.
Adding generative artificial intelligence (GAI)
Large language models (LLM’s), a building block of Chat GPT, are essentially highly advanced, probabilistic, auto-fill capability based upon training data from the entire internet. Further enablement by the cloud and massive computing will accelerate the learning process, and asymptotically approach perfection––but only for the reporter. In the context of a unified IT stack, once the unification extends to the other touchpoints of the radiology enterprise and its partners, the learning capabilities will extend by orders of magnitude.
Text is just one form of data we deal with. Historically, the output (voice recognition) component of text is totally under the radiologist’s control and oversight. On the other hand, the input of important data (images, patient data, etc.) depends on other sources. Radiologists have long been protagonists of the “garbage in, garbage out” narrative. No more! GAI’s pathway will present curated pre-interpretation information and enhanced post-analysis reports and recommendations, respectively.
With the advent of big data analytics, natural language processing, and machine learning, the unified stack becomes a powerhouse. Patient history, demographics, laboratory results, operative notes, and genetics data combine to provide a comprehensive narrative for each case and to each care provider, readily available at the time of report creation. This empowers radiologists and makes their input more meaningful to the treating physicians (and vice versa).
Supporting value-based, patient-centered care
As healthcare increasingly focuses on the value provided to patients, the ability to communicate and interpret comprehensive patient information, integrating disparate components, becomes paramount. Patients gain the same access to reports with hyperlinked images as every other stakeholder. Instead of numbers on a page (series 4, image 37), patients can click the link and see exactly what their care team is talking about. This unified vision plays a pivotal role in enhancing the overall value delivered to patients.
Additionally, GAI helps radiologists cross-reference comorbidities in a way that was not possible before. For instance, people with certain types of autoimmune arthritis have an increased risk of cardiovascular disease (atherosclerosis, hypertension, and type 2 diabetes). These conditions might seem unrelated, but if a CT scan reveals calcifications in the coronary arteries, GAI can facilitate informing the radiologist and treating physician of this important biomarker. These types of added value are not just consumer conveniences. As potentiators of clinical research and effectuators of episodes of care, they can save the lives of patients.
Leaning into the whole
It should be clear to most in the industry that AI is knocking at the door, and those who do not adopt new technology will be left behind. What seems less clear is how that design and implementation should move forward. Laying AI functions on top of already outdated systems or relying on separate solutions that do not play into the unified stack system––especially given the volume of data, delicate privacy issues and the need for constant updates––does not optimally contribute to advancement. Instead, we should embrace the vision as a whole and build for unification and GAI, rather than jury-rig a square peg in a round hole.
Conclusion
The path of radiology has been marked by remarkable achievements, but the journey is far from over; we are on the cusp of an exhilarating transformation that promises improved patient outcomes, elevated care delivery, and a brighter, unified future.
As we look ahead, maximizing value hinges on integrating the new tools available to us with a holistic approach to the care continuum. By enhancing radiologist efficiency and effectiveness, streamlining information flow, and integrating various components of the radiology ecosystem, then amplifying the system with GAI, we can unleash healthcare knowledge in a way that reshapes patient care delivery. Instead of the goal being enterprise imaging, we will be a prototype in the pursuit of Enterprise Knowledge.
Dr. Alan Kaye has broad leadership experience in many facets of radiology and health care. He is a past president of the American College of Radiology after having served on several ACR Commissions, including Economics, Government Relations, Information Technology and the Future Trends Committee. Dr. Kaye served as President & CEO of an innovative, high growth private practice in Connecticut, and has held leadership positions at a large community hospital, residency program, multispecialty physician organization, and national health insurer advisory panels. He has been an appointee to numerous Connecticut state health care task forces, committees, and advisory panels. Dr. Kaye believes Sirona’s novel RadOS platform will best positively impact radiologists’ pivotal role in patient care.