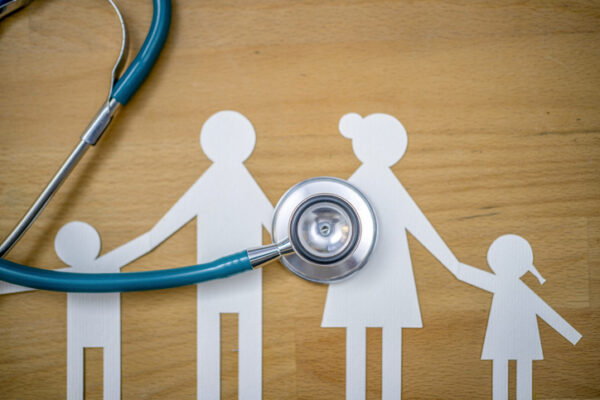
The implementation of AI is becoming table stakes, with use cases and successes in healthcare becoming a reality. However, as the industry shifts the AI narrative from hype to cautious adoption, it necessitates a look beneath the surface, which is uncovering big questions around safety, governance and tangible impact.
The usual concerns about the technology persist, including bias, sources of training data, misinformation, and hallucinations. Because of this, caution remains – and rightly so. The next step for AI is to show that it can bridge the gap between its data-founded, theoretical world and the complexities of live patient care where consequences are real and sometimes life and death.
This leaves us in a predicament where we need to continue the development of the technology for the purposes of improving outcomes, while also insulating patients and providers from unexpected, negative outcomes. Guardrails and watchful eyes need to be standard as we continue to come up with a technology that can consistently provide appropriate outcomes.
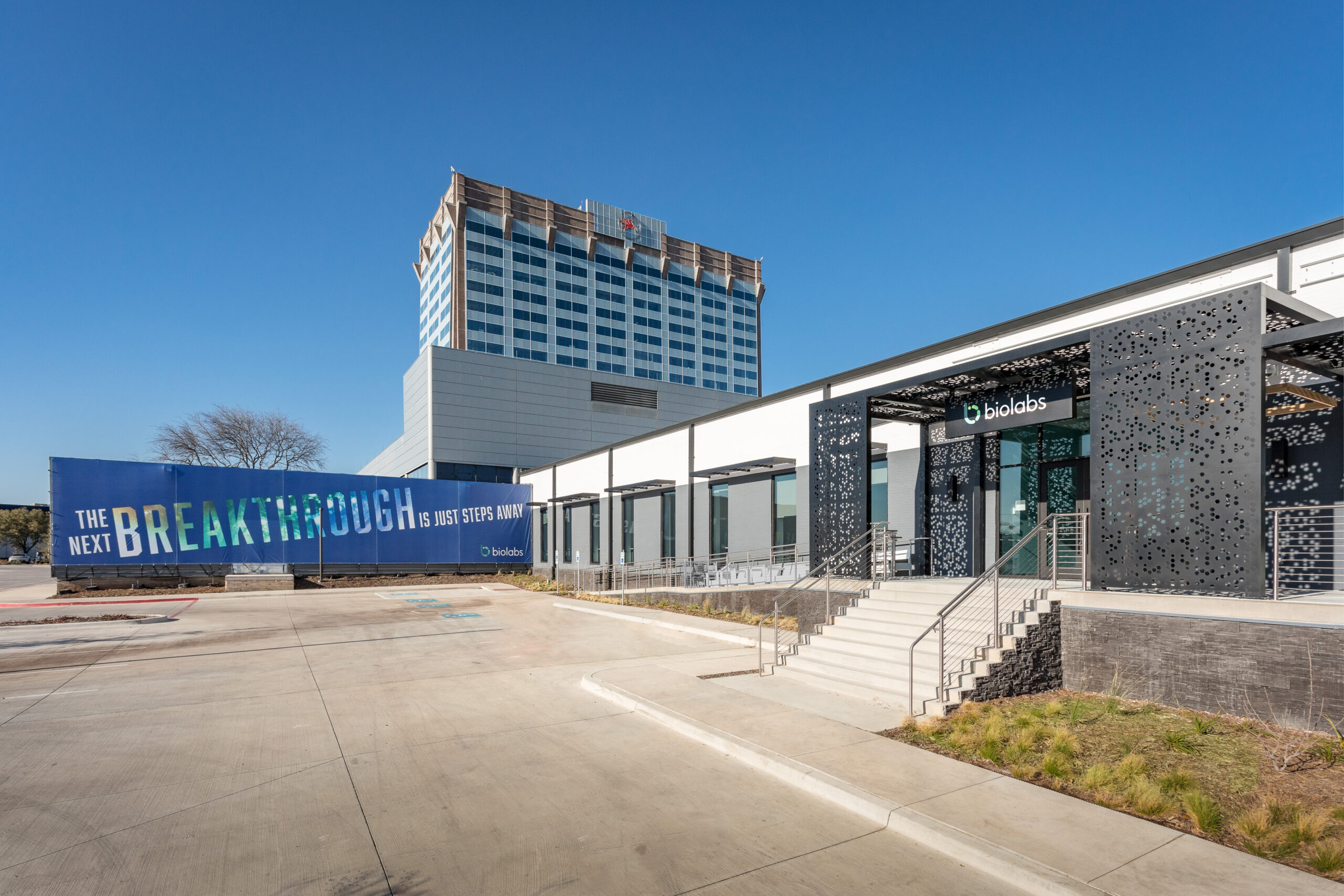
BioLabs Pegasus Park Cultivates Life Science Ecosystem
Gabby Everett, the site director for BioLabs Pegasus Park, offered a tour of the space and shared some examples of why early-stage life science companies should choose North Texas.
The right inputs for the right outputs
Many health systems are eager to utilize AI, as it’s already delivering on its promise in a number of clinical areas by reducing providers’ workloads and improving performance.
For instance, AI solutions are expediting clinical documentation workflows by capturing and recapping provider-patient conversations, and saving providers hours of administrative work that’s usually done at home. AI models are also used to enhance diagnostic imaging, streamlining the discovery-to-diagnosis process, and identify patient risk factors. Some providers are even using ChatGPT to aid with research and help them communicate empathetically with patients.
However, there have been inconsistencies across outcomes, stressing caution over hype. For instance, one study might find poor outcomes for ChatGPT (users asked 39 medication-related questions to ChatGPT, and the model provided accurate responses to about a quarter of them; the rest were incomplete or inaccurate, and it did not address some questions), while another showed that ChatGPT passed the USMLE.
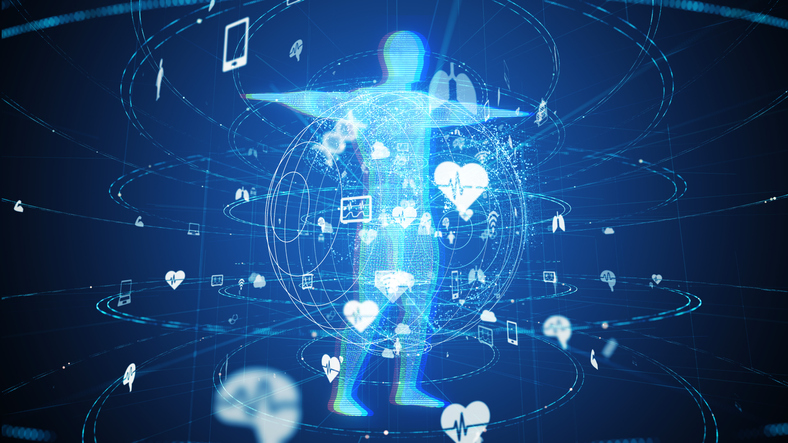
With the Rise of AI, What IP Disputes in Healthcare Are Likely to Emerge?
Munck Wilson Mandala Partner Greg Howison shared his perspective on some of the legal ramifications around AI, IP, connected devices and the data they generate, in response to emailed questions.
These results highlight the lapse in taking AI at face value. There is no room for these types of errors or inconsistencies in the precise world of medicine. Not only does AI need healthcare-specific training to avoid using public and unreliable sources, but the AI needs to be trained, managed and, most importantly, used the right way. There is no reason, at this time, that an AI should be functioning in healthcare without some form of human guidance.
Humanizing AI training for specificity
AI has proven it can have an immediate and safe impact as a co-pilot for providers. This works because it’s here that AI can function with high accuracy, have the greatest impact, and see acceptance from providers. By including a human in the mix, AI can shine. AI will only be as good as the humans and the data that it is trained on. There are bound to be biases and inaccuracies that bleed through from training, but by adding more trained eyes to the mix before we deliver outputs to patients, we add collective checks and balances that reduce these issues.
The aphorism of “garbage in, garbage out” is critical in any AI use case—meaning if we train AI models with low-quality data or a lack of guidance, we’ll receive low-quality outputs. This becomes even more important in clinical use cases. While every model should be trained with proven medical data and internal data from health systems and providers, looping in human providers will allow for more specific insights into how the AI should operate, providing better patient interaction, a heightened ability to assess a situation and more accurate outputs.
The benefits of a provider feedback loop
When AI models have a provider feedback loop, everything becomes more accurate, as is expected. The feedback loop acts as a built-in review stage where direct input from specialists and supervising providers can teach AI models about the intricacies and nuances of patient care.
The feedback loop has been used extensively in the Japanese national healthcare system, in over 1,500 clinics and hospitals to empower doctors for better patient care. It consists of patients using the tool for intake, which then feeds directly into a provider facing dashboard. This equips providers with a foundation for a conversation, allowing them more time to focus on empathetic care and crafting a personalized treatment plan. Moreover, it offers guidance on differential diagnosis that ensures doctors consider possibilities of rare and orphan diseases. At the end of the appointment, the provider assesses how well the AI functioned and confirms if their diagnosis matches the AI’s predictions, providing valuable feedback that ensures accuracy improves over time.
With a provider feedback loop, the benefits stretch beyond speed to diagnosis:
- AI goes from theory to practice: When providers are involved in AI training, they fill the gap between theory and practice. Importantly, this goes beyond the data, which may or may not improve the function of an AI. Humans can provide insights through more detailed context than a machine can, thereby bringing the AI closer to the accuracy of an actual human.
- Providers trust the technology: Providers understandably only accept AI if it’s proven, of high quality, and demonstrates the ability to improve patient experiences. Technology partners, in turn, must have a vision that matches up with clinical validation. That’s how providers become champions or evangelists of the technology. Trained-by-them AI tools also give providers agency in the technology, as it’s working with them, not trying to replace them.
- Patients have better experiences: One study found that patients are more satisfied with their doctors when interactions aren’t rushed, when the doctor has a caring, friendly demeanor, and when they listen and ask for patient input. Conversely, most patients dislike it when providers spend too much time entering information on their computers rather than speaking to them.
With the right solutions, such as those that provide symptom insights before the appointment to doctors or help capture conversations in real-time, providers can spend more time with the patient, building the relationship, earning their trust, and focusing on the forward-looking health journey.
AI technologies have great promise within clinical care, but they cannot learn the intricacies and nuances of patient care on data and numbers alone. The humanization of AI, specifically through a provider feedback loop, is one of the key ways to elevate these solutions and to increase trust and adoption with health systems, providers, and patients.
Photo:boonchai wedmakawand, Getty Images
Kota Kubo graduated from the University of Tokyo Graduate School of Engineering. In 2013, while enrolled at the University of Tokyo, he began researching and developing software and algorithms that simulate the relationship between doctors, symptoms and disease names. He worked at M3 Corporation for about three years, working on software development and web marketing in the BtoC healthcare field, including doctor Q&A services. In 2017, he founded Ubie with his co-representative doctor Abe.