Machine learning promises to dramatically improve the efficiency and effectiveness of healthcare, bringing us closer to the kind of personalized medicine that not only can substantially improve preventive care, but also bring the right treatment to the right individuals at the right time. We’re seeing growing application in medical imaging analysis, along with tools that use artificial intelligence to improve medication adherence and follow-up care.
However, when it comes to predicting, diagnosing and treating medical conditions, many are still skeptical. The concerns are multi-faceted:
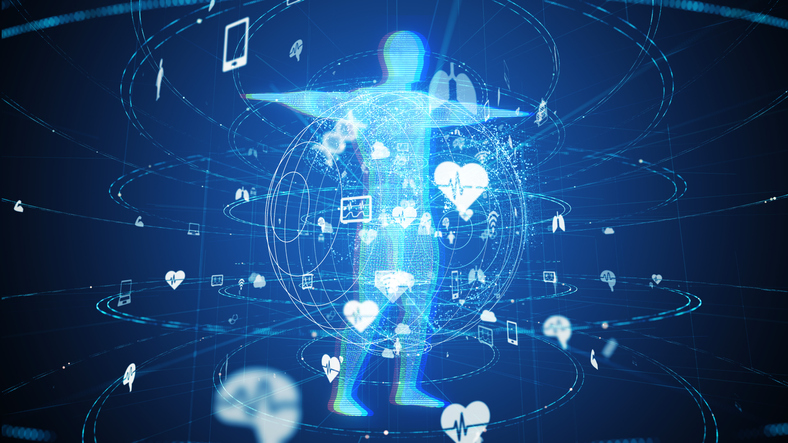
With the Rise of AI, What IP Disputes in Healthcare Are Likely to Emerge?
Munck Wilson Mandala Partner Greg Howison shared his perspective on some of the legal ramifications around AI, IP, connected devices and the data they generate, in response to emailed questions.
Data quality
As with any analytics solution, the quality of the results is only as good as the quality of the data the system has to work with. Small sample sizes, “dirty” or incomplete data and biased data can all impact the analysis, which could result in skewed conclusions. In this case, data-driven mistakes could mean the difference between life and death for gravely ill patients or those with multiple confounding conditions.
Manipulation risk
Not only could the data be unintentionally flawed, there is also the risk that it could be intentionally manipulated. Either the data or the neural networks that “teach” the machine learning algorithms could be programmed to introduce bias or lead clinicians to false conclusions. While it’s hard to imagine anyone acting maliciously in this way, it’s not out of the question, nor is the risk of manipulating data to show more favorable results of treatment protocols or drugs.
Obscured logic
Because of the inherent risks, physicians and other clinicians need to understand why and how machine learning solutions arrive at their conclusions. Black box algorithms that spit out recommendations without explanation or insight into their reasoning create more questions than answers. This lack of transparency naturally leads to skepticism in a field where so much expertise relies on inherent physician experience.
Given these limitations, will we ever trust machine learning models in medical applications? What will it take for machine learning to deliver accurate, reliable conclusions and recommendations?
Here are four factors that should be present for improving accuracy and overcoming skepticism and risk:
Confidence scores
Rather than just issuing a conclusion or prediction, machine learning models must accompany that result with a confidence score—the probability that the suspected condition is associated with other known data. This helps to determine the result that is most likely correct and gives clinicians an opportunity to review results with the highest confidence scores against what he or she knows about the case or has observed with the patient. Confidence scoring helps to overcome the “black box” problem by giving clinicians insight into the reasoning process behind the output.
Complex rules
Some machine learning determinations are based on one-to-one associations, such as if/then correlations. Applying complex machine-learned rules, in which multiple factors are considered in making a prediction, can dramatically improve the accuracy and confidence level of the output. Intuitively, it makes sense that results based on multiple pieces of data are naturally more thorough and accurate; therefore, models that use 3-to-1 rather than 1-to-1 rules would provide greater confidence in the outcome. Furthermore, exclusionary criteria (eliminating conditions a patient is known NOT to have) can also increase validity and accuracy.
Clinical data
Most machine learning models rely on administrative or claims data — primarily billable coded conditions and prescriptions. However, there is a tremendous amount of valuable insight in clinical data, diagnostic report notes and physicians’ exam notes. For example, a suspected diagnosis of unspecified heart failure based on prescribed medication and other coded evidence may only have a confidence score of 70 percent. But, the accuracy and confidence would be substantially improved if evidence of diastolic dysfunction on an echo report, volume overload in an X-ray report or a physician’s observation/notation of edema were added into the equation. The ability to pull this into the machine learning analysis can dramatically improve accuracy and confidence in the output.
Natural Language Processing
Unstructured data, like physician’s notes and diagnostic reports, comprise about 80 percent of patient information, but getting that into the machine learning algorithm is extremely difficult. Using a sophisticated Natural Language Processing (NLP) engine that understands human language can bring that data into analysis. By processing physician narratives through a library of words, concepts and relationships, NLP engines can understand not just the individual words but also the context behind a collection of words to capture the meaning. NLP engines designed specifically for clinical language (as opposed to legal language, for example) significantly improve NLP accuracy. We can even apply machine learning to the NLP itself, enabling the engine to become smarter by analyzing new data from coders and physicians to refine its understanding of grammar patterns and generate new rules to optimize accuracy.
Machine learning is a powerful tool that can help clinicians understand and discover new clinical associations among patient populations to refine preventative care and treatment protocols. However, understanding its limitations is critical—it is a tool, not a solution. There’s no substitute for a skilled physician’s expertise in considering the unique clinical situation of each patient. With the right data and approach in place, however, machine learning can certainly help to accelerate diagnosis, treatment and the development of effective preventative programs. This will not only improve the quality and efficiency of care for both individual patients and broad populations, but also increase clinician and facility productivity, allowing care providers to treat more patients more effectively.
Photo: ANDRZEJ WOJCICKI, Getty Images