For years, health researchers have been talking about the potential of real-world data (RWD) to revolutionize every stage of clinical research, from trial design to outcomes measurement. Healthcare systems track hundreds of millions of patient touchpoints each year, recording this information in the form of electronic health records (EHR), insurance claims, and other clinical systems that represent an incredibly rich source of data and insight. So, if the industry is so excited by this revolution, why hasn’t it been more widely adopted in clinical research?
The primary reason is that RWD has been largely unavailable to clinical trial teams, hindered by patient privacy, regulatory restrictions and disparate data structures. While there have been notable successes in using available EHR and claims data, deeper and more valuable clinical data has been inaccessible. Liberating the genetics, laboratory and pharmacy data that sits deep within healthcare subsystems, while protecting patient information and navigating the global regulatory landscape, is no easy task. But considering the upside of getting real-world evidence (RWE) right, the effort is more than worth it.
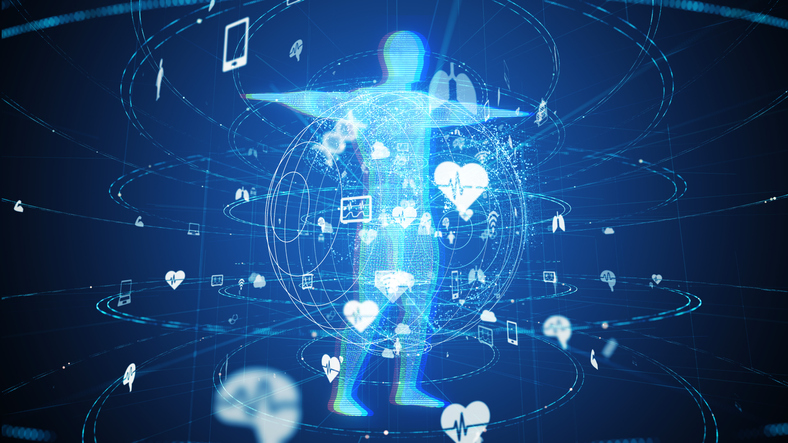
With the Rise of AI, What IP Disputes in Healthcare Are Likely to Emerge?
Munck Wilson Mandala Partner Greg Howison shared his perspective on some of the legal ramifications around AI, IP, connected devices and the data they generate, in response to emailed questions.
The real-world data gap within clinical trials
There is no doubt that both RWD and RWE are playing an increasing role in healthcare decisions and research. Both are used regularly to monitor post-market safety and adverse events. According to a presentation given by Medidata at Reuters Event Pharma: Clinical 2021, RWD also plays an essential role in regulatory approvals for new indications: 75% of new drug applications and biologic license applications in 2020 included evidence from RWD. Many hospitals have adopted a “learning health system” model to track and analyze data for research and continuous improvement. Conversely, most clinical trials are conducted in a “one-off” manner, without a way to draw on the treasure trove of efficacy and safety signals that sit within the ecosystem of healthcare data.
Using RWD within an RCT to develop and validate new treatments is the natural next step in the evolution of healthcare delivery and clinical research. And the potential for data use begins long before the approval phase—with opportunities to enrich virtually every step of the clinical trial journey.
Cohort identification and enrichment
The use of RWD can help to optimize trial arms by identifying more homogeneous and targeted patient populations. The data might enable the clustering of cohorts with similar profiles, who have responded in the same way to a particular drug or have biologic signals pointing to similar likelihood of disease progression. Artificial intelligence can help to dig deeper into clinical datasets that are often siloed, including biomarkers, labs, images, transcriptomes, proteomes and genomes, enabling more precise study designs to vet treatments.
This deep data can also illuminate trends that can be utilized for predictive enrichment of trial populations. The ability to target cohorts who are more likely to respond positively to a drug may allow researchers to run smaller clinical trials, while potentially generating more rapid and decisive clinical efficacy and safety outcomes. For example, evidence has shown that cancer patients with the HER2 genetic mutation don’t respond to platinum-based chemotherapies. The ability to leverage RWD to retrospectively ‘screen’ cancer patients for HER2 genetic mutations and to track the progression of disease amongst these patients, could enable the identification of patient subgroups that would be excellent targets for an alternative non-platinum treatment.
Patient recruiting
One of the leading barriers in many clinical trials is the ability to meet enrollment quotas in a sufficient timeline to prevent trial closure. While there is a general awareness that untapped patient pools exist, a map of where to find them and their providers has remained elusive. Use of RWD and the mining of deep datasets are poised to provide access to these hard-to-find patients, and could be particularly useful for finding patients with rare diseases, rare cancers, and cancers with specific genetic mutations.
In addition, deep data mining could enable the pre-identification of patients likely to become drug resistant to the standard of care. These patient pools could then be contacted and ready for next-line therapies in available clinical trials.
Lastly, there are disease states in which the standard of care is no intervention, because the patients whose conditions are likely to progress represent too small a fraction of the total population. Deep mining of RWD can find the profile of the known progressors and make predictions about who are most, versus least, likely to endure disease progression by mapping multifactorial profiles that are not only based on patient disease state, but also on treatments, prescriptions and geo-demographics.
Of course, access to health insights should never come at the expense of patient privacy. RWD is typically anonymized by generating a unique code (token) for each patient to protect their identity while enabling teams to aggregate across disparate datasets. However, there are situations where it can be beneficial to associate data with a specific patient—for example, to offer access to a clinical trial in a way that respects the patient’s privacy.
Patient and physician engagement
In a growing number of cases, patients are successfully recruited by leveraging the relationship between them and their HCPs. But to accomplish this, providers need to be made aware of trial opportunities that would suit their patients. RWD can play a key role in identifying relevant cohorts and informing their HCPs of trial opportunities. Providers can then advise and accompany their patients through the trial process. New partnerships between researchers and health providers have the potential to deliver a win-win for all parties, aligning research and care delivery around the common goal of improving patient outcomes.
Physicians can:
- Access a broader array of studies that could meaningfully improve their patients’ health
- Conduct trial activities within their existing point-of-care workflows
- Screen, diagnose and recommend patients for potential study inclusion
- Preserve continuity of care and maintain revenue by overseeing patients’ medical assessments throughout the trial
- Conduct federated research with fellow providers, expanding the impact of their work
Meanwhile, partnerships with providers allow researchers to benefit from the doctor-patient relationship by:
- Identifying pre-screened participants who fit study criteria
- Recruiting and enrolling patients who are more likely to be engaged in the study
- Improving compliance with the study
At the end of the day, the general public is the ultimate beneficiary of provider-researcher partnerships. Expanding the pipeline for clinical trials will connect more patients with life-changing treatments.
Expanding clinical trials through control arms
In addition to identifying suitable participants, RWD can also provide historic or synthetic control arms. These external arms can minimize patient exposure while enabling novel therapeutic and/or interventional medical device candidates to emerge at a more rapid and efficient pace. By tracking the progression of patient populations outside a trial who fit the inclusion and exclusion criteria and are not receiving a drug, researchers can in some cases avoid having to recruit a control population into the trial. An important patient benefit of this approach is that all those in the trial receive the drug versus placebo. RWD also enables the use of existing datasets to conduct meta-analyses to understand novel indications and/or side effects that may not be revealed in any one clinical trial due to insufficient numbers.
There are a few promising cases where an external control has helped achieve drug approval: RWE was used to support effectiveness decisions for BAVENCIO, Pfizer’s immunotherapy for metastatic merkel cell carcinoma and BLINCYTO, Amgen’s treatment for B-cell precursor acute lymphoblastic leukemia. Both received accelerated approval using data from external, historical controls.
Streamlined data aggregation and the continuum of care
RWD can also help to improve outcomes measurement in clinical research by facilitating the integration of EMR, remote patient monitoring (RPM) and electronic patient-reported outcomes (ePRO) data for analysis. Here too, inefficient data extraction has historically been a major barrier to achieving this potential and again, new forms of partnerships are breaking down these barriers.
For example, collaboration between organizations conducting decentralized clinical trials and healthcare and diagnostic software solutions providers can help to connect the dots between clinical research and EHR data.
Such partnerships facilitate the integration of data from multiple sources under the umbrella of a single clinical trial, including:
- Pseudo-anonymized meta-data insights from EHRs
- Medical records (including laboratory tests, imaging, scans and clinician notes) from consenting patients
- RPM data (e.g., from wearables and other devices) and ePRO from these same patients when they are study participants
Innovative partners within the industry are developing a unique approach to distributing data queries and running AI models “at the edge” (without having to move the data to a central repository) to ensure data privacy while enabling federated data access. With patient and provider consent, these collaborations will also allow for the mapping of EHR data into the Clinical Trial Case Report Form system used by research teams to create a more robust record of each trial participant. Some partners are also working to move beyond traditional claims data, using machine learning to integrate deeper data sets such as clinical notes, labs, images, and biomarkers typically not available in existing data sets.
The end benefits of real-world data for sponsors
Leveraging real-world data allows sponsors and CROs to improve quality, control and cost-effectiveness along the full research cycle, by:
- Designing targeted trials offering a more comprehensive view of patient populations, cohorts and disease states
- Accelerating patient recruitment and improving compliance through provider partnerships
- Creating synthetic control arms
- Conducting richer, more efficient analyses by identifying correlations and disease progressions (for instance, studying connections between childhood and adult diabetes)
- Identifying new or expanded indications for the study product
- Enhancing regulatory dossiers with credible third-party data to improve approval prospects
The advantages of integrating RWD into clinical trials extend far beyond any single study. The broader window into patients’ health may enable researchers and providers to better predict disease onset and offer more informed treatment and care recommendations.
Of course, as with any tech innovation, significant challenges remain to greater use of RWD in clinical trials: Integration of disparate and complex data sets with no common language, formatting, or coding can require sophisticated programming to ensure data currency, accuracy and minimize bias. Systems must safeguard absolute data privacy / anonymization or integrate careful consent management, taking into consideration the different regulatory standards across regions (GDPR, HIPAA, PDPA). And then there are the scientific challenges: How to put the necessary quality controls in place when creating synthetic arms to ensure that outcomes are valid and representative?
Despite these challenges, there is general consensus in the industry that RWD will be integral to the future of clinical trials, with the potential to deliver more robust findings and increase the ROI of health innovation. The onus is on the research community to develop the necessary guardrails, collaborations and tools to deliver less hype and more results.
Photo: RomoloTavani, Getty Images
Clarification: An earlier version of this article could have been misconstrued as suggesting that RWD data would be used to exclude rather than to protect certain populations with co-morbidities.
For over 25 years, Susan Dallabrida has served as a leader in clinical trials, healthcare, and eClinical technology for biopharmaceutical and health-related consumer goods companies. Her career began at Harvard Medical School—where she served as Principal Investigator and Lab Director at the Children's Hospital—before she transitioned into new roles with Biogen, ERT, Oracle Health Sciences. Dallabrida holds a doctorate from Pennsylvania State University, College of Medicine, with a Ph.D. in Biochemistry and Molecular Biology. At ObvioHealth, Susan leads rater, patient, and caregiver training, as well as ePRO, ClinRo, and instrument development and validation.
Craig Gravina has over 25 years’ experience developing highly innovative platforms, products, and disruptive technologies within healthcare and life sciences. He has managed several successful developments and launches across the industry, including a digital therapy platform for Leso Digital Health, cognitive assessment solutions at Cogstate, and an analytics and population health platform and portfolio at Allscripts. In his work with ObvioHealth, Craig and his team have architected a next-generation healthcare platform and introduced innovative product capabilities across the venture portfolio.