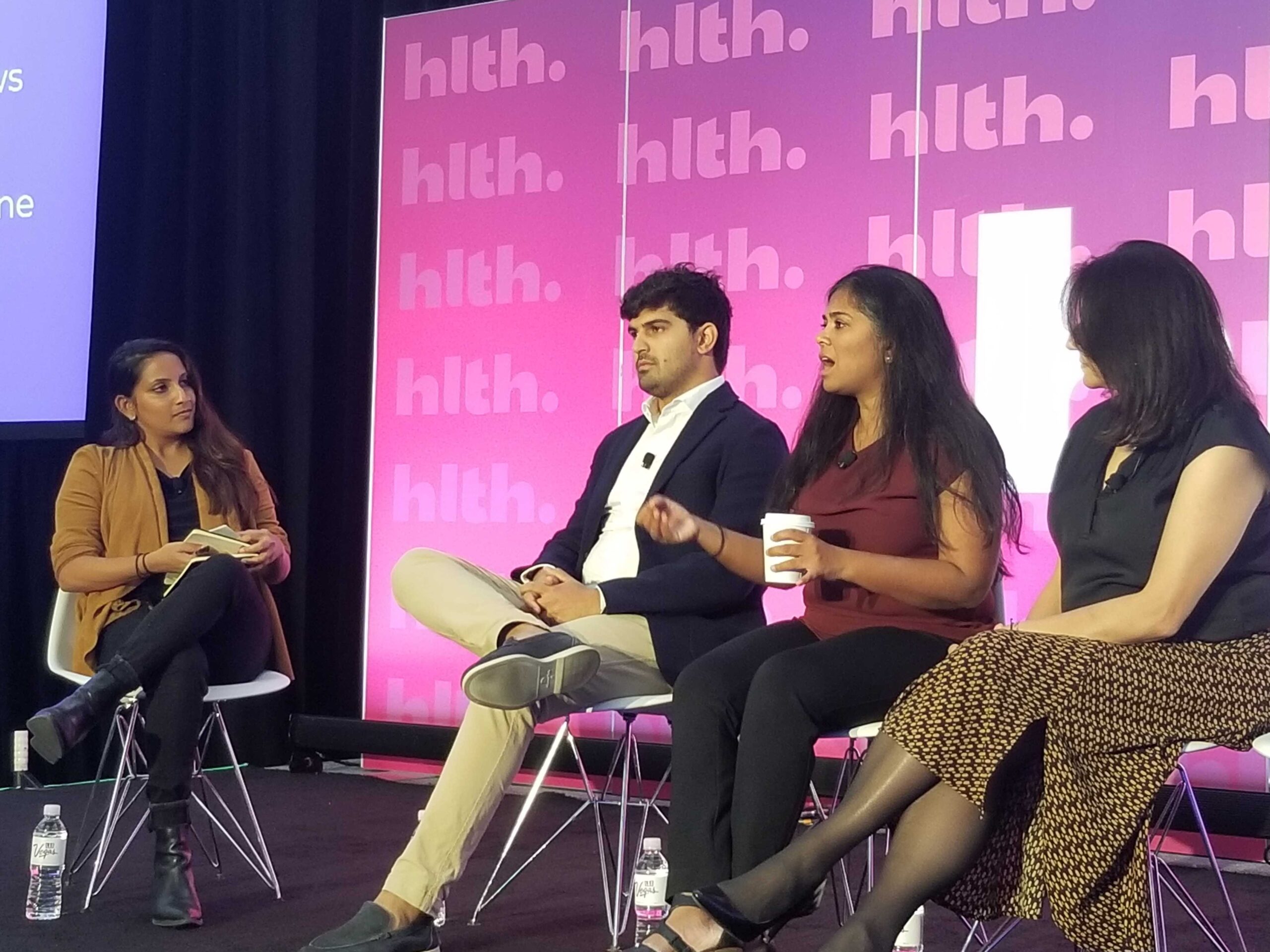
From left to right: Mohana Ravindranath, STAT News’ Bay Area correspondent; Alex Momeni, partner at General Catalyst; Suchi Saria, CEO at Bayesian Health; and Sunita Mishra, chief medical officer at Amazon Health Services
Healthcare, like most other industries, has been experimenting with the use of large language models (LLMs) over the past year to streamline things like patient communication, clinical documentation and prior authorization.
While there has certainly been a great deal of hype recently surrounding LLMs and other forms of generative AI, there has also been a lot of skepticism. When it comes to an industry like healthcare, where one small technology failure could be the difference between life and death, many stakeholders are concerned about how to mitigate the risks associated with novel LLMs entering the field.
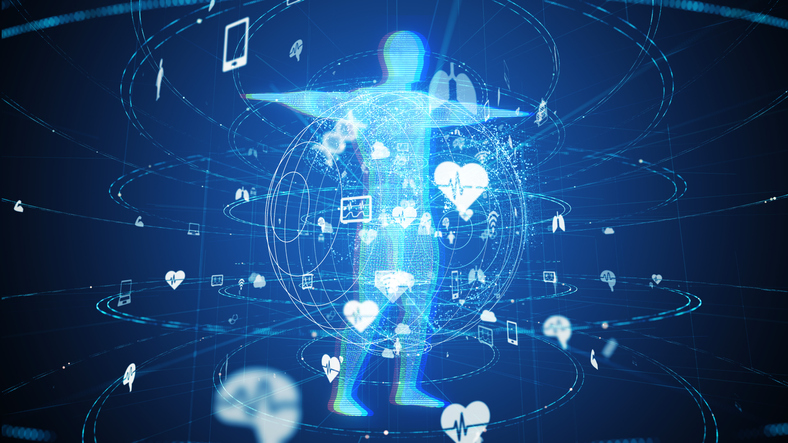
With the Rise of AI, What IP Disputes in Healthcare Are Likely to Emerge?
Munck Wilson Mandala Partner Greg Howison shared his perspective on some of the legal ramifications around AI, IP, connected devices and the data they generate, in response to emailed questions.
The use of LLMs in healthcare is still quite nascent, so the industry lacks a cohesive, shared framework for regulating the use of these AI models. But healthcare technology experts have confidence that the sector will be able to put the right guardrails up as it continues to develop and deploy LLM tools, they said Sunday during a panel discussion at Engage at HLTH in Las Vegas.
To illustrate this confidence, General Catalyst Partner Alex Momeni drew a comparison between cars and LLMs.
“We’ve had cars for a very long time. Cars have different parts, including the engine. So if we say the AI model is the engine, there are actually a lot of things you can build around it — you can figure out how to solve the safety issues in a practical way. We invented seat belts and airbags over time — we made cars way safer for passengers. With AI, the challenge is how we create an environment where we iterate to figure out what some of the problems are and then put guardrails around it and not expect the model alone to be perfect,” he explained.
Because the LLMs hitting the healthcare scene are so novel, many leaders are focusing their deployment on administrative use cases and staying away from clinical applications, which they can view as more risky. But Suchi Saria, a machine learning researcher at Johns Hopkins and CEO of Bayesian Health, pointed out that this type of thinking can be flawed.
Some of the administrative use cases being explored by providers are patient-facing. For example, LLMs can be used to help patients pay their medical bills or triage their symptoms at home. Just because these tools don’t help doctors make clinical decisions doesn’t mean that they are without risk, Saria noted. If an autonomous patient-facing LLM malfunctions or misinterprets a piece of data, it could potentially be dangerous — for example, a LLM-powered chatbot could tell a person who needs to go to the emergency department that they can manage their condition at home.
“With anything that is fully autonomous, you have to think about what the guardrails around it are,” Saria cautioned.
And ultimately, establishing strong safety guardrails will help build both provider and patient trust in LLMs, she pointed out. A great way to build trust is through accountability, she added.
Saria recalled a conversation she had recently with a leader from a provider organization that had just started using ChatGPT to generate patient messages. The organization was grappling with whether they should disclose that these messages were written by an LLM or say that they were coming from the patient’s physician. The provider decided that it should tell patients the messages are sent by physicians — this decision created a sense of responsibility that “makes sure that the providers are being accountable for what’s being sent out,” Saria explained.
This choice mitigates the risk of physicians’ automation bias because it ensures they are cognitively engaged with the tool and checking its content for accuracy before sending it out to patients, she noted.
Photo: Stephanie Baum, MedCity News