Earlier this week, the Office of the National Coordinator for Health Information Technology released the winners of its Patient Matching Algorithm Challenge.
The purpose of the contest, which was announced in May, was to encourage the development of algorithms that match patients with the correct information.
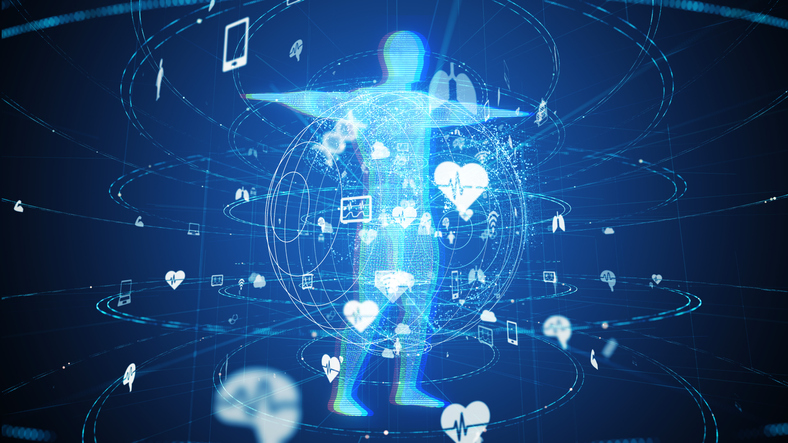
With the Rise of AI, What IP Disputes in Healthcare Are Likely to Emerge?
Munck Wilson Mandala Partner Greg Howison shared his perspective on some of the legal ramifications around AI, IP, connected devices and the data they generate, in response to emailed questions.
The challenge is an attempt to tackle a continuing healthcare problem: aligning the patient data held by one provider with the same patient’s data held by another. The issue makes interoperability all the more difficult and can put patient safety at risk.
“Many experts across the healthcare system have long identified the ability to match patients efficiently, accurately and to scale as a critical interoperability need for the nation’s growing health IT infrastructure,” said Don Rucker, the ONC’s national coordinator for health IT, according to a blog post. “This challenge was an important step towards better understanding the current landscape.”
Using an ONC-provided dataset, more than 140 teams sent in nearly 7,000 submissions. But the agency managed to drill them down to a first, second and third place winner. The competitors were judged based on the best “F-score,” which measures accuracy in terms of precision and recall.
Palo Alto, California-based Vynca nabbed the top spot, securing a prize of $25,000. PICSURE came in second, earning $20,000, and Information Softworks got third place and $15,000.
Additionally, PICSURE won the “best recall” and “best precision” awards, as well as the $5,000 prize that accompanies each of them. The “best first run” award and $5,000 went to Information Softworks.
ONC noted that the companies utilized different approaches, such as an algorithm based on the Fellegi-Sunter method and a stacked model method.
Photo: FotografiaBasica, Getty Images